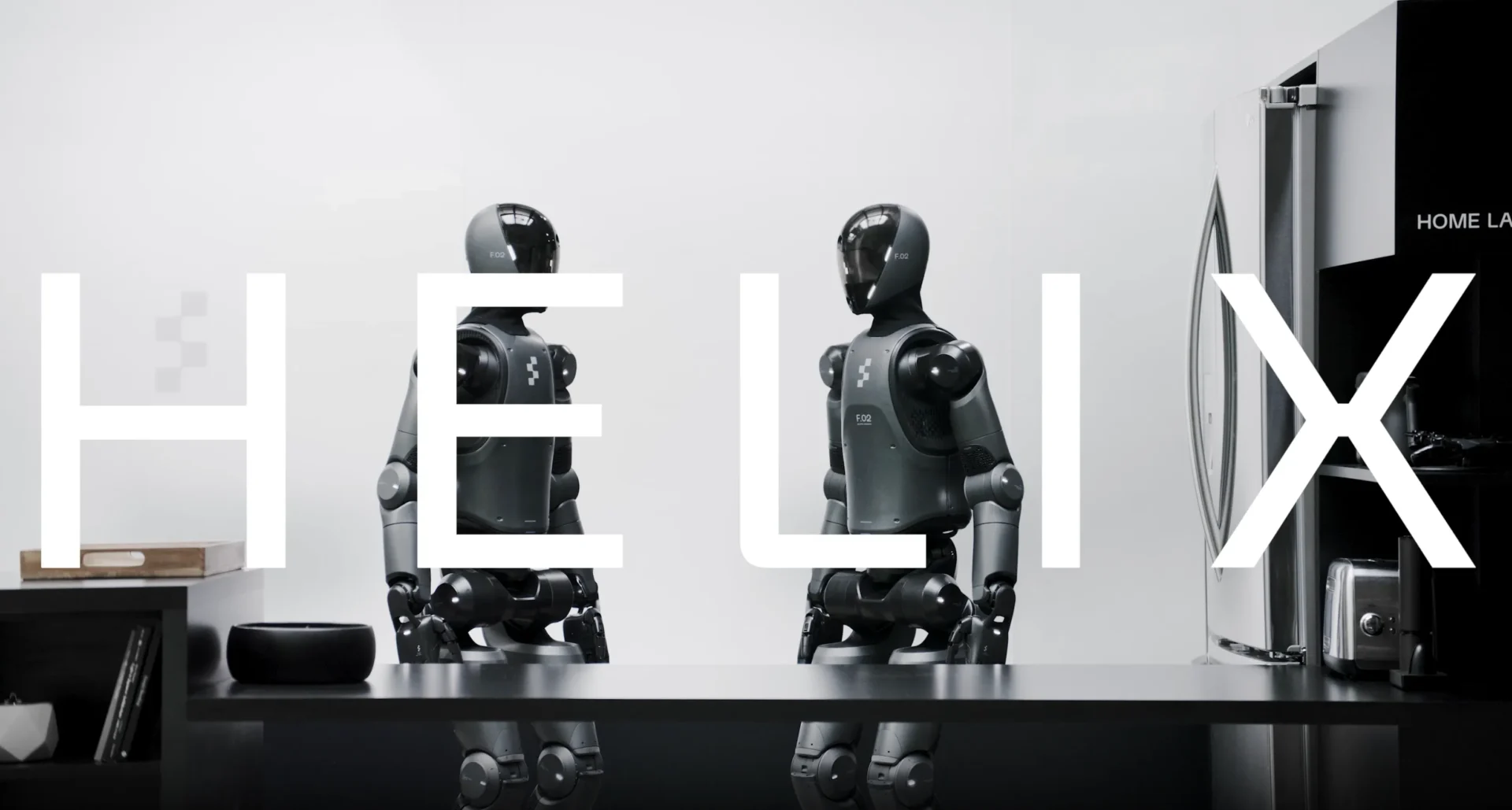
Figure AI Helix: Revolutionary Vision-Language-Action Model for Robotics
Figure AI has just introduced Helix, its new Vision-Language-Action (VLA) model, and it could be a game-changer for robotics. Designed to help robots better understand the world around them, follow human instructions, and take real actions, Helix shows major promise — especially for home environments.
In a recent demo, Figure’s humanoid robots, powered by Helix, successfully completed a classic household task: putting away groceries. It sounds simple, but it’s a big deal in robotics. The robots picked up items they had never seen before — like an onion and a bag of shredded cheese — and placed them neatly into a fridge or cabinet. They figured out (pun intended) where everything belonged without any human help. Even more impressive? Two robots worked together to get the job done, showing off Helix’s ability to enable multi-robot teamwork.
Wondering where the actual video is? We’ve got you!
How Does Helix Work?
At its core, Helix is built to translate what a robot sees and hears into what it does. It combines two neural networks — one that handles slower, more thoughtful decision-making and another that reacts quickly to immediate changes. This combination lets the robot reason through complex tasks but still respond to its environment in real-time.
Unlike many AI systems that rely on heavy cloud computing, Helix runs entirely on low-power GPUs built right into the robot. That means these robots don’t need a constant internet connection and are efficient enough for real-world use.
Inside Helix: How It’s Built and Trained
Helix isn’t just another AI model — it’s designed from the ground up for real-world robotics. To get there, Figure AI used a combination of deep learning, imitation learning, and an architecture tailored for physical interaction.
1. Vision-Language-Action (VLA) Model
Helix is part of a new wave of VLA models that blend three key AI capabilities:
- Vision: The robot perceives its environment using cameras and depth sensors, turning raw data into an understanding of objects, spaces, and relationships.
- Language: It interprets human commands using natural language processing (NLP), allowing it to follow instructions like “put the milk in the fridge.”
- Action: Finally, it maps this combined understanding into real-world movements, controlling its arms, hands, and even its walking gait to complete tasks.

2. Dual Neural Networks: Thinking vs. Reacting
To make the system more flexible and reliable, Helix uses two separate neural networks:
- Reasoning Network: This slower, high-level network handles planning and decision-making. It figures out what needs to happen — like recognizing a grocery item and deciding where it should go.
- Reactive Control Network: This faster, low-level network takes care of moment-to-moment control, ensuring the robot can adjust on the fly to changes, like a slipping object or an unexpected obstacle.
This split lets the robot balance complex reasoning with quick reflexes, similar to how humans can both plan ahead and react instinctively.
3. Training: Learning by Imitation
Helix was trained using around 500 hours of teleoperated behaviours, where human operators remotely controlled the robots to complete a wide range of tasks. This process, known as imitation learning, teaches the model by example — essentially letting the robot “watch and learn” from humans.
Here’s how the process worked:
- Teleoperation Sessions: Humans performed tasks like opening doors, grasping fragile objects, and navigating around obstacles.
- Data Collection: Every action, sensor reading, and environmental variable was recorded.
- Neural Network Training: The data was fed into the networks, allowing Helix to generalize from these examples and start handling new, unseen situations.
Imitation learning is especially effective for robotics because it helps models avoid the trial-and-error pitfalls of traditional reinforcement learning, where robots can often “break things” during training
4. Hardware Optimisation
A key feature of Helix is that it runs entirely on embedded, low-power GPUs. This keeps the energy requirements down and makes the robots more autonomous — they don’t need to constantly ping cloud servers for help, reducing latency and potential downtime.
This hardware setup also makes Helix more scalable. Because it doesn’t require massive computing resources, multiple robots can run Helix simultaneously, as seen in the demo where two humanoids collaborated to put away groceries.
Is It Ready for Everyday Use?
While the demo was undeniably impressive, experts are urging some caution. Dr. Kostas Bekris, a robotics professor at Rutgers University, called the demo an “exciting development” but pointed out that the setup was pretty ideal — the groceries were placed neatly, and there weren’t any obstacles. “The real challenge is seeing if it can handle messier, less predictable situations,” he said.
Figure AI trained Helix using about 500 hours of teleoperated behaviours, where humans remotely controlled the robots to teach them a variety of tasks. This kind of imitation learning is common in robotics, but it’s not without its limitations — especially when moving from a demo environment to the real world.
Dr. Christian Hubicki from Florida State University echoed these concerns, noting that “reliability remains the primary stumbling block for general-purpose robots.” He emphasized that while demos are exciting, consistency in real-life situations is key.

What’s Next for Figure AI?
Figure AI has been making waves in the robotics world. Last year, the company raised $675 million and even signed its first commercial deal with BMW, bringing its robots into automotive production lines. Now, with Helix showing promise for home environments, Figure seems to be setting its sights on consumer markets.
But before we see Helix-powered robots organizing pantries or folding laundry in our homes, there’s still work to be done — especially when it comes to testing the robots in more complex, everyday scenarios.
For now, though, it’s hard not to get excited about the possibilities. If Helix can truly handle the unpredictability of real life, it could be a major step toward the kind of helpful home robots we’ve been dreaming of for decades.
You May Also Like
DeFi 3.0 – The Next Generation of Decentralized Finance Applications and Infrastructure
Ut enim ad minim veniam, quis nostrud exercitation ullamco laboris nisi ut aliquip ex ea commodo con
The Hidden World of AI: Prompt Engineering and Jailbreaking
Ut enim ad minim veniam, quis nostrud exercitation ullamco laboris nisi ut aliquip ex ea commodo con
Top 5 AI & ML Trends Shaping Tech organizations in 2024
Ut enim ad minim veniam, quis nostrud exercitation ullamco laboris nisi ut aliquip ex ea commodo con