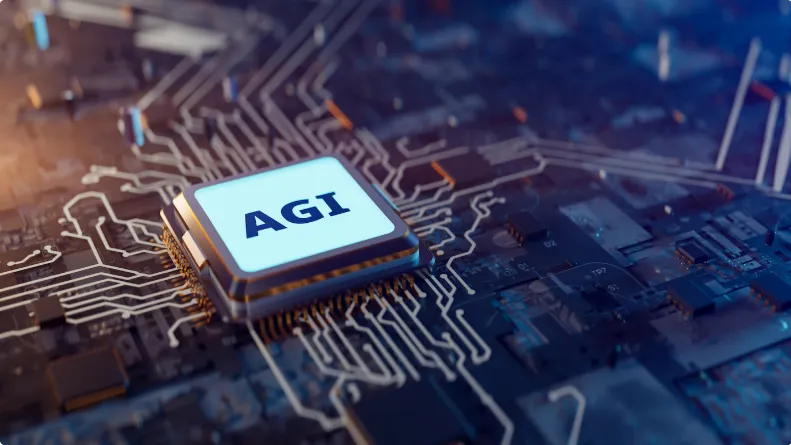
AGI: The Quest for Artificial General Intelligence
Artificial intelligence (AI) has revolutionised how we interact with technology, from voice assistants to complex data analysis tools. However, the current generation of AI, known as narrow or weak AI, is designed for specific tasks. The ultimate goal for many researchers and tech companies is to create Artificial General Intelligence (AGI) — a form of AI that can perform any intellectual task that a human can, demonstrating adaptability, learning ability, and general problem-solving skills. This blog will delve into what AGI is, its current state of research, the companies leading the way, and the challenges and implications of its development.
What is AGI?
Artificial General Intelligence (AGI) refers to AI systems that possess the ability to understand, learn, and apply knowledge across a broad range of tasks at a human-like level. Unlike narrow AI, which is designed to handle one specific task (e.g., language translation or image recognition), AGI would have the cognitive capabilities to perform diverse activities, adapt to new environments, and reason about complex concepts without task-specific programming.
AGI systems are envisioned to:
- Generalise across domains: Perform well across different types of tasks without needing significant reprogramming.
- Learn efficiently: Adapt and learn from minimal data inputs, similar to how humans do.
- Reason and problem-solve: Make decisions that involve abstract thinking and context comprehension.
The Current State of AGI Research:

Despite its promise, AGI is still largely theoretical and considered an open challenge in the field of AI. Current machine learning models and neural networks are powerful but lack the cognitive flexibility and depth that AGI requires. Here’s an overview of where the research stands:
- Advances in Machine Learning and Deep Learning: While modern machine learning models such as GPT-4 (by Openai) and other sophisticated neural networks can produce impressive outputs, they operate under the principles of pattern recognition and predefined training data. They do not understand tasks in the way humans do; rather, they excel at statistical prediction.
- Breakthroughs in Neuroscience and Cognitive Science: AGI research often draws on insights from neuroscience to mimic how the human brain works. Understanding human cognition — how we learn, store information, and generalise knowledge — continues to be a guiding force in developing AGI algorithms.
- Progress in Cross-Domain Transfer Learning: Transfer learning allows AI to apply knowledge gained from one domain to a different, albeit related, task. This is seen as a stepping stone to AGI, as it hints at more flexible learning capabilities that resemble human intelligence.
Companies Leading the AGI Race:
Several prominent tech companies and research institutions are at the forefront of AGI research, aiming to push the boundaries of what AI can achieve:

1. OpenAI
- Key Contributions: OpenAI, known for its work on GPT models (including ChatGPT), has AGI as a long-term goal. The company aims to develop a safe AGI that benefits all of humanity. Their work in large language models is seen as a foundational step toward AGI, as these models are capable of performing a wide array of tasks with surprising accuracy and adaptability.
- Mission Statement: OpenAI emphasises the ethical development of AGI, focusing on safety, transparency, and the fair distribution of its benefits.
2. DeepMind (Owned by Alphabet Inc.)
- Breakthroughs: DeepMind’s AlphaGo, AlphaFold, and other projects have demonstrated the potential of combining deep learning and reinforcement learning. Their recent work involves creating more generalised models, with projects like Gato, a multi-modal model designed to perform different tasks such as image recognition, dialogue, and robotic control.
- Philosophy: DeepMind’s research often merges AI with human-level cognitive processes, inspired by theories in psychology and neuroscience.
3. Anthropic
- Background: Founded by ex-Openai employees, Anthropic focuses on AI safety research. The company’s approach involves creating more interpretable and controllable AI models, with AGI safety being a top priority.
- Initiatives: Research on robust decision-making frameworks and AI alignment, which are critical for safe AGI deployment.
4. IBM Research
- Current Projects: IBM’s Watson platform laid the early groundwork for cognitive AI, although it remains far from AGI. IBM’s research now focuses on AI that can reason more like a human through projects in neuromorphic computing and explainable AI.
- Vision: IBM aims to create AI systems capable of more autonomous decision-making, crucial for achieving AGI.
5. Microsoft and Openai Partnership
- Joint Ventures: Microsoft’s significant investment in Openai underlines its commitment to the development of AGI. This partnership enables OpenAI’s cutting-edge research to be integrated into real-world applications via Azure’s cloud computing capabilities.
Challenges in Developing AGI:
While the idea of AGI is appealing, its development comes with significant challenges:
1. Technical Hurdles
- Data Efficiency: Current AI models often require vast amounts of data for training. AGI, however, would need to learn and generalise from much less data.
- Computational Power: The computational resources needed to train and run AGI models are orders of magnitude greater than what is used for today’s AI systems.
2. Safety and Alignment
- Ethical Concerns: One of the most debated aspects of AGI is ensuring it aligns with human values and ethical standards. An AGI that can learn and act autonomously poses risks if it does not adhere to safe and controlled behaviour.
- Control Mechanisms: Researchers are developing frameworks to ensure AGI behaves predictably and can be stopped or redirected if necessary. This involves work in AI alignment and explainability.
3. Philosophical and Societal Implications
- Impact on Jobs: The widespread implementation of AGI could dramatically change the job market, leading to debates on universal basic income and job re-training programs.
- Existential Risks: Prominent voices, including the late Stephen Hawking and Elon Musk, have warned about AGI potentially surpassing human intelligence and becoming uncontrollable if not carefully managed.
The Future Outlook for AGI
The journey toward AGI is expected to be long and fraught with complex challenges, but it holds extraordinary promise. As AI models grow in their ability to understand and replicate human cognition, researchers are hopeful that AGI will unlock new solutions in fields ranging from healthcare and education to environmental sustainability.
Key Takeaway: While we are still in the era of narrow AI, the advancements being made today are setting the stage for more general, adaptable systems that inch us closer to AGI. The progress by companies like OpenAI, DeepMind, and others is a testament to the immense effort and dedication needed to reach this next frontier of artificial intelligence.
In conclusion, AGI stands as one of the most profound challenges in modern science and technology. Whether its development will bring unprecedented benefits or unforeseen risks depends on the actions and decisions taken by the pioneering companies and the research community today.
You May Also Like
Agentic AI: The Next Frontier in Artificial Intelligence
Ut enim ad minim veniam, quis nostrud exercitation ullamco laboris nisi ut aliquip ex ea commodo con
Cloud Solutions for Startups
Ut enim ad minim veniam, quis nostrud exercitation ullamco laboris nisi ut aliquip ex ea commodo con
Top 5 AI & ML Trends Shaping Tech organizations in 2024
Ut enim ad minim veniam, quis nostrud exercitation ullamco laboris nisi ut aliquip ex ea commodo con